There are increasing concerns about data privacy and online security around the world; this is somewhat of a paradox, as users continue to give away personal data (and thus their privacy) in exchange for different services. A recent survey [CIGI-Ipsos 2019] on Internet security and trust found that 78 percent of Internet users in 25 economies were at least somewhat concerned about their privacy online. Internet scams of various types have also been demonstrated to raise internet users’ sensitivity to privacy issues [Chen 2017]. While economic development theory has long grappled with the consequences of cross-border flows of goods, services, ideas, and people, the most significant growth in cross-border flows now comes in the form of data. Some of these flows represent ‘raw’ data while others represent high-value-added data; this can make a difference in the trajectory of national economic development [Weber 2017]. Public awareness about privacy risks on the Internet is increasing; with the evolution of the Internet to the Internet of Things, these privacy risks are likely to become even more significant due to the large amount of data collected and processed by IoT architectures [Baldini 2018]. The Sony pictures hack[1] illustrates that privacy is not just an individual concern; unease over privacy expectations has emerged at the individual, governmental and international levels. Conceptually and methodologically, privacy is often confounded with security. [Spiekerman-Hoff 2012]. Gartner expressed a concern that the biggest inhibitor to IoT growth will be the absence of security by design[Gartner 2018] (which would include some aspects of privacy). While there has been considerable attention placed on some aspects of security, privacy has received less attention from the IoT community. Privacy was identified this year by Deloitte[2] to be the factor driving regulatory uncertainties over data management. This regulatory uncertainty challenges enterprises’ adoption of new technologies (like blockchain, or IoT). Social expectations for privacy are evolving, particularly in regard to aggregate representations of personal data in cyberspace. IoT devices and architectures are emerging as a major new data source for capturing representations of human activity. Rising cyberspace privacy concerns are moving beyond isolated activities like web browsing or social networks to consideration of the privacy impacts of the aggregate representation of personal data, including foreseeable data generation capabilities of IoT architectures. At a minimum, this creates a public relations problem for the deployment and operation of IoT Architectures.
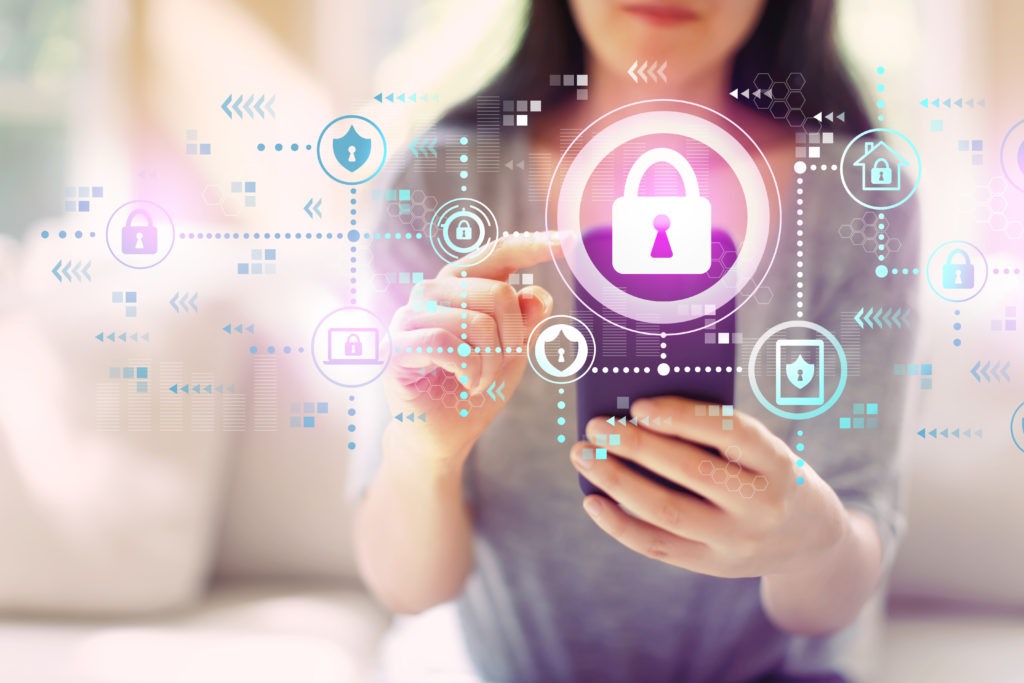
IoT networks, like many other networks, are not technically constrained within geographical or political boundaries, but these political constructs may imply legal obligations for participants. Many of these legal notions of privacy evolved prior to the availability of the internet. International treaties like the UNDHR [UN 1948] and ICCPR [UN 1976] provide some definitional guidance on privacy rights, and [ALI 1977] identifies US common law privacy torts related to intrusion upon seclusion, appropriation of name or likeness, and publicity given to private life. These legal concepts, however, were all in place before the deployment of the Internet and the emergence of IoT. US legal requirements on privacy also come from a variety of other sources including constitutional limits, legislation, regulation, common law, and contract law; while litigation processes like discovery also implicate privacy. The Federal Trade Commission provides some cross-industry-sector privacy enforcement, but other industry-specific agencies in the health, finance, education, telecommunications, and marketing enforce industry-specific privacy regulations. States have also promulgated their own laws (e.g., on data breach notification and reporting obligations). [Solove 2006] proposed a privacy taxonomy with four main groups of activities that threated privacy (1) information collection (including surveillance and interrogation); (2) information processing (including aggregation, identification, insecurity, secondary use and exclusion); (3) information dissemination (including breach of confidentiality, disclosure, exposure, increased accessibility, blackmail, appropriation, and distortion); and (4) invasions (including intrusions and decisional interference). More recently, the General Data Protection Regulation [EU 2016] (GDPR) applies extraterritorially to protect EU citizens and has also been influential in other national privacy efforts. In particular, GDPR identifies roles in managing data (e.g., Data Protection Officers); rights for data subjects (including breach notification, access to their personal information, data erasure (the right to be forgotten), and data portability); and requires privacy to be incorporated into the design of systems (Privacy by Design). Globally, privacy laws are continuing to evolve towards bringing greater rights to data subjects [Greenleaf 2019]. Legal considerations on privacy generally revolve around the rights and obligations of legal entities; the IoT, however, is generally considered from the perspective of “things” and the data they generate or consume. The “things” in IoT are not usually considered legal entities, but many recent proposals for IoT architectures have been based on blockchains, and some have argued that blockchains could be implemented as Digital Autonomous Organizations (DAOs) structured to be recognized as independent legal entities (e.g., zero-member LLCs [Bayern 2014] or BBLLCs [Vermont 2018]). Manufacturers of IoT systems often seek the scale of global markets, and so cannot avoid these international trends in privacy regulation. IoT architectures have historically not emphasized privacy features, or considered IoTs operating as independent legal entities. The threats of increased regulation and the opportunities of new legal options will challenge existing IoT deployments and create opportunities for new IoT architectures.
The data we collectively create and copy each year is growing at 40% annually is estimated[3] to be around 44ZB/yr in 2020 (that’s around 6TB/yr for every person on earth), with much of this data expected (in future) to come from IoT devices sensing the world around them. Today, while people may choose to consume their portion of all their data as internet cat videos, many are not mindful of the digital footprints they leave in cyberspace [Camacho 2012]. An entirely new value chain has evolved around firms that support the production of insights from data. Individual data are worth very little on their own; the real value of data comes from the data being pooled together. [Beauvisage, 2017]. IoT provides a major new source of data for the big data value chain. Beyond intentional internet interactions, IoT sensor networks can also passively collect data on human activities. At the earlier stages of the data value chain, information content is limited, and therefore the scope for value generation is also low; at the same time, the data is more personalized and hence more susceptible to privacy threats. Some types of data should not be extracted, for instance, if it impinges on fundamental privacy rights. Some data, such as health data, may be usefully extracted under highly regulated circumstances. For many IoT architectures, the privacy threat arising from information processing (e.g., aggregated data) may be more severe than individual data samples. IoT data does not have to be as bandwidth-intensive and focused as video surveillance to threaten privacy. Patterns of private human activity can be discerned from aggregating data from disparate IoT architectures. The ownership and control options for IoT architecture generated data (as relating to human privacy) may be more complex than previous IoT architectures had considered – perhaps rather than centralizing data from IoT sensors in the cloud, IoT data may need to remain distributed, but responding to a limited set of authorized queries. Some actors may also have access across multiple IoT architectures providing a further degree of information aggregation and processing. Even IoT architectures intended for other purposes (e.g. environmental monitoring) may have the data they generate repurposed in ways that violate human privacy. For IoT architectures to succeed in large scale commercial deployments, they must be prepared to address evolving privacy concerns. This will require IoT architecture to identify which of the data they generate can implicate human privacy concerns.
Humans are interacting with vast amounts of data in new and unusual ways. Sensor density in consumer products is also increasing. Cyberspace historically was just an environment in which computer communication occurred; now it is already defined more by users’ social interactions rather than technical implementation concerns [Morningstar 2003]. Cyberspace computation today is often an augmentation of the communication channel between real people. People seek richness, complexity, and depth within a virtual world; and at the same time require increasing annotation of real-world entities with virtualized data in augmented reality. Humans increasingly use cyberspace for social interaction merging cyberspace and social spaces into social computing. The environments, however, are not the same; humans’ expectations and intuitions from the physical world do not always carry over into cyberspace. For example, real-world experiences are ephemeral; thanks to data storage, however, representations of personal data do not naturally decay; applying this to privacy violations, a transient real-world peeping incident equivalent in cyberspace could result in an ongoing data peeping threat. Legal notions of privacy are typically sensitive to the context (e.g., public spaces vs homes) and actors (e.g., people, organizations, governments). If IoT deployment scale projections are correct, then cyberspace in the near future will be dominated by data flows from IoT architectures. Cyberspace may create notions of new types of entities that may implicate privacy [Kerr 2019], and DAOs are one example of this. Devices are evolving to provide more “human-like” interfaces (e.g. voice assistants (e.g. Alexa, Siri) AI chatbots [Luo 2019]) and autonomous activity (e.g. UAV drones, Level 5 self-driving cars). The Apple iPhone 11 sensors include[4] faceID, barometer, three-axis gyro, accelerometer, proximity sensor, ambient light sensor, audio, and multiple cameras. The Tesla Model 3 includes[5] rear side and forward cameras, forward-facing radar and 12 ultrasonic sensors. The increasing data intensity in human experience is affecting human behavior and perceptions. While data generically is a very abstract concept, IoT sensor data is very much concerned with creating and aligning various linkages between physical reality and its cyberspace counterpart. Many actors may have an interest in the data about humans created by IoT devices and architectures. Beyond data ownership considerations, recent privacy legal initiatives have created new roles and additional obligations for operators of IoT architectures – e.g. GDPR’s rights to correct data or to be forgotten. The scope, scale, and serendipity of individual human interactions with cyberspace are reaching a qualitative change as IoT architectures become more pervasive.
The human-computer interaction (HCI) with the IoT blockchain is also an important factor affecting whether privacy enhancements are successful. Click through licenses can easily permit users to contract away their privacy rights (unless otherwise limited by regulation). There have been some efforts[6] to provide better exemplars of legal patterns for privacy information; adoption, however, is voluntary unless there is some superseding regulation (e.g., requiring specific notices to “opt-in” for certain types of information disclosures). Given the evolving nature of privacy concepts, HCI approaches may be helpful [Wong 2019] to better define users’ perceptions of the privacy problem space. Trademarks and certification seals may be useful [Wirth 2018], [Bansal 2008] for consumers to identify and trust products and services that provide privacy assertions (e.g., conformance to privacy regulations such as the GDPR). Beyond disclosures, new privacy rights create functions (e.g., for authorized modification or deletion of data) that need to be supported in IoT architectures. The effectiveness of such functions in providing humans with more advanced controls of their personal data will depend in large part on their ease of use. The usability/ operability of such user controls of their data will also be impacted by the visibility and accessibility of the privacy controls. IoT use cases need to evolve to consider these new roles and functions within IoT architectures and how humans can effectively use them to maintain control of their privacy.
Two fundamental technology trends are driving the Internet of Things (IoT). Firstly, the continued miniaturization of devices through Moore’s law, nanotechnology, new materials, etc., is providing an increased density of functionality in devices, and a consequent increase in the variety and volume of the data these devices can generate and consume. Secondly, the number and quality of connections are increasing. Gartner estimated[7] there would be 8.4 billion connected Internet-of-Things (IoT) devices in use worldwide in 2017 and projects an increase to 50 billion by 2020. IoT use cases are one driver for 5G deployments and these deployments are also expected to increase connectivity density towards ubiquity in many areas. Ericsson estimates[8] there will be 1.5 billion IoT devices with cellular connections by 2022 with cumulative annual growth rates on the order to 20%-30%. This is significantly faster growth than the US GDP growth (estimated[9]in the range 2-3% 2018-2019) or world population growth rates (estimated[10]at 1-2%). Even the job outlook for software developers is only expected[11] to improve by ~21% (2018-2018). The number of IoT devices and their connectivity is evolving the Internet to be primarily an Internet of Things, where the IoT devices, and the data they communicate, forms the dominant usage pattern. This massive IoT investment comprises multiple information infrastructures; forming a cyberspace data environment within which people will interact for an increasing portion of their lives. With massive IoT deployments expected within the next 5 years, to avoid stranded investments, it is important to get the appropriate IoT architecture requirements in place to address common human concerns, particularly around privacy. Existing IoT deployments will also be impacted by privacy as public relations headwinds, evolving regulatory requirements on management of IoT data, changing human attitudes due to the qualitative changes in cyberspace experiences from pervasive IoT environments, and increased user control of IoT data. Retrofitting privacy (or security) into an existing distributed architecture is unlikely to be simple cheap or complete. New IoT architectures must consider privacy impacts.
References
[ALI 1977] American law Institute, “Restatement of the law, Second, Torts”, 1977, § 652
[Baldini 2018] G. Baldini, et al. “Ethical design in the internet of things.” Science and engineering ethics 24.3 (2018): 905-925.
[Bansal 2008] G. Bansal, et.al., “The moderating influence of privacy concern on the efficacy of privacy assurance mechanisms for building trust: A multiple-context investigation.” ICIS 2008 Proceedings (2008)
[Bayern 2014] S.Bayern, “Of bitcoins, Independently wealth software and the zero member LLC”, Northwestern U.Law Rev. vol 108, pp 257-270, 2014
[Beauvisage 2017] T. Beauvisage (2017). Selling one’s behavioral data: An impossible market? (Research blog). Orange. April 18. Available at: https://recherche.orange.com/en/selling-ones-behavioral-data-an-impossible-market/.
[Camacho 2012] M.Camacho, et. al., “Self and identity: Raising undergraduate students’ awareness on their digital footprints.” Procedia-Social and Behavioral Sciences 46 (2012): 3176-3181.
[Chen 2017] H.Chen, et.al., “Securing online privacy: An empirical test on Internet scam victimization, online privacy concerns, and privacy protection behaviors.” Computers in Human Behavior 70 (2017): 291-302.
[CIGI-Ipsos 2019] CIGI-Ipsos, UNCTAD and Internet Society (2019). 2019 CIGI-Ipsos Global Survey on Internet Security and Trust. Centre for International Governance Innovation, UNCTAD and the Internet Society. Available at: https://www.cigionline.org/internet-survey-2019.
[EU 2016] European Union: Regulation (EU) 2016/679 of the European Parliament and of the Council of 27 April 2016 on the protection of natural persons with regard to the processing of personal data and on the free movement of such data, and repealing Directive 95/46/EC (General Data Protection Regulation)
[Gartner 2018] R.Contu, et.al.,“Forecast: IoT Security, Worldwide, 2018”, Gartner, Tech. Rep., 2018.
[Greenleaf 2019] G. Greenleaf, “Global Data Privacy Laws 2019: New Eras for International Standards.” (2019).
[Kerr 2019] Kerr, Ian. “Schrödinger’s Robot: Privacy in Uncertain States.” Theoretical Inquiries in Law 20.1 (2019): 123-154.
[Luo 2019] Luo, Xueming, et al., “Frontiers: Machines vs. Humans: The Impact of Artificial Intelligence Chatbot Disclosure on Customer Purchases.” Marketing Science (2019).
[Morningstar 2003] C.Morningstar, et. al., The Lessons of Lucasfilm’s Habitat. The New Media Reader. Ed. Wardrip-Fruin and N. Montfort: The MIT Press, 2003. 664-667.
[Solove 2006] Daniel J. Solove “A Taxonomy of Privacy”. U. Pa. L. Rev., 154:477–560, 2006.
[Weber 2017] S. Weber, “Data, development, and growth.” Business and Politics 19.3 (2017): 397-423.
[Spiekerman-Hoff 2012]. S.Spiekermann-Hoff, “The challenges of privacy by design.” Communications of the ACM (CACM) 55.7 (2012): 34-37.
[UN 1948] United Nations, “Universal Declaration of Human Rights”, 1948
[UN 1976] United Nations, “International Covenant on Civil and Political Rights”, 1976
[Vermont 2018] Vermont S.269 (Act 205) 2018 §4171-74
[Wirth 2018] C. Wirth, et. al., “Privacy by blockchain design: a blockchain-enabled GDPR-compliant approach for handling personal data.” Proc. of 1st ERCIM Blockchain Workshop. European Society for Socially Embedded Technologies (EUSSET), 2018.
[Wong 2019] R. Wong,
et.al., “Bringing Design to the Privacy
Table: Broadening ‘Design’ in ‘Privacy by Design through the lens of HCI” Proceedings
of the 2019 CHI Conference on Human Factors in Computing Systems. ACM,
2019.
[6] https://bit.ly/33vyyzt