Autonomy is relevant in many different activities; and has recently received a lot of attention in the context of autonomous mobility of cyber-physical devices. A broad range of mobile physical devices are being developed for a variety of different geographic environments (Land, Sea, Air). These devices have various capabilities of internal controls often described with a ‘Levels of Autonomy’ taxonomy. Autonomous mobility is largely concerned with the problems of navigation and avoidance of various hazards. In many cases, these autonomous mobile devices are proposed as potential replacements for human operated vehicles which creates some uncertainty regarding potential liability arrangements when devices have no human operator. The levels of autonomy proposed for autonomous mobility might provide some insight for levels of autonomy in other contexts e.g., DAOs.
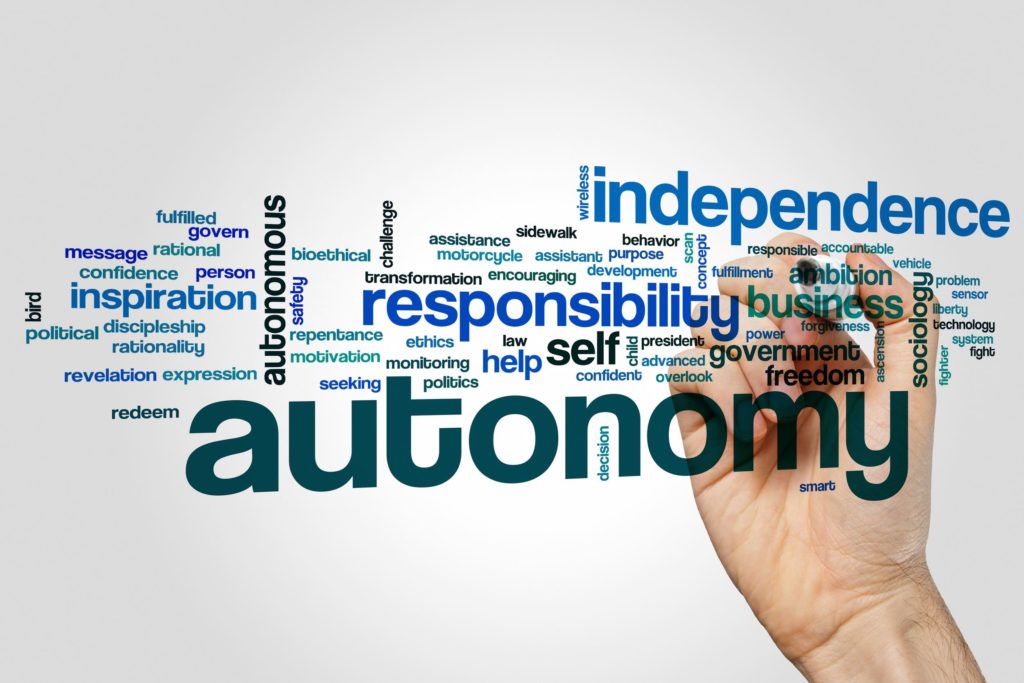
Levels of Land Mobile Device Autonomy
Automobile-specific regulations and more general tort liabilities have established a body of law where the driver is in control of the vehicle, but this is challenged by automation culminating in autonomous vehicles [Gasser 2013]. In their more recent review of autonomous vehicles, [Taeihagh 2019] categorized technological risks and reviewed potential regulatory or legislative responses in US and Europe; where the US has been introducing legislations to address autonomous vehicle issues related to privacy and cybersecurity; While the UK and Germany, in particular, have enacted laws to address liability issues; other countries mostly acknowledge these issues, but have yet to implement specific strategies. Autonomous vehicles are expected to displace manually controlled vehicles in various applications becoming an increasingly significant portion of the vehicular traffic on public roads over time. [SAE2018] Defines 6 levels of automation:
- Level 0 – No Driver Automation – May provided momentary controls (e.g. ,emergency intervention).
- Level 1 – Driver Assistance – Sustained, specific performance of a subtask
- Level 2 – Partial Driving Automation – sustained specific execution with the expectation that the driver is actively supervising the operation.
- Level 3 – Conditional Driving Automation – sustained, specific performance with the expectation that the user is ready to respond to requests to intervene as well as to performance failures
- Level 4 – High Driving Automation – Sustained, specific performance without the expectation that the user will respond to a request to intervene
- Level 5 – Full Driving Automation – Sustained an unconditional performance without any expectation of a user responding to a request for intervention
Autonomous vehicles on off-road applications add another layer of complexity for route planning, steeper slopes, hazard recognition, etc. [Elhannouny 2019]. The levels of autonomy for land mobile autonomous systems in this the taxonomy are sequenced as decreasing levels of human supervision.
Levels of Sea Mobile Device Autonomy
Maritime autonomous systems pose many additional challenges to their designers as the environment is more hostile and the device may be more remote than land mobile autonomous systems etc. Without a well-defined safety standards and efficient risk allocation, it is hard to assign liability and define insurance or compensation schemes leaving unmanned surface ships potentially subject to a variety of different liability regimes [Ferriera 2018]. Unmanned maritime systems have been in operation for more than 20 years [Lantz 2016] and more frequent interactions between manned and unmanned systems are expected in future. The International maritime Organization [IMO 2019] recently initiated a regulatory scoping review of the conventions applicable to Maritime Autonomous Surface Ships. The Norwegian Forum for Autonomous Ships [NFAS 2017] proposed a four-level classification of autonomy:
- Decision Support – Crew continuously in command of vessel
- Automatic – pre-programmed sequence requesting human intervention when unexpected events occur or when sequence completes
- Constrained Autonomous – fully automatic in most situations but human operators continuously available when requested by the system
- Fully Autonomous – no human crew or remote operators.
As with land-based mobility autonomy, the levels of this taxonomy for sea mobile autonomous systems are defined and sequenced as decreasing levels of actions by human operators.
Levels of Air Mobile Device Autonomy
The air environment adds an additional degree of freedom (vertical) for navigation, and introduces different navigation hazards (e.g. birds, weather) compared to land or sea environments. Human operated air mobile devices have in the past been relatively large, expensive and heavily regulated. Smaller lower cost drones have enabled more consumer applications. In the US, the FAA has jurisdiction over drones for non-recreational use [FAA 2016], though an increasing number of states [NACDL 2019] are also promulgating regulations in this area. Potential liabilities include not just damages to the device, but also liabilities from collisions with fixed infrastructure, personal injuries, trespass, etc.
[Clough 2002] proposed a 10-level classification of autonomous control levels:
- Level 0 – remotely piloted vehicle
- Level 1 – execute preplanned mission
- Level 2 – Pre-loaded alternative plans
- Level 3 – Limited Response to Real Time Faults/ Events
- Level 4 – Robust response to anticipated events
- Level 5 – Fault/ Event adaptive vehicle
- Level 6 – Real Time Multivehicle Coordination
- Level 7 – Real Time multi vehicle cooperation
- Level 8 – Multi-Vehicle mission performance optimization
- Level 9 – Multi-Vehicle tactical performance optimization
- Level 10 – Human Like
More recently, [Huang 2005] proposed autonomy levels for unmanned systems along three dimensions: Mission complexity, Human Independence and Environmental difficulty. The levels of this autonomous air mobility taxonomy are defined and sequenced as increasing complexity of the scenario and its implied computational task.
Consolidated views of mobility autonomy levels
[Vagia 2019] reviewed levels of automation across different transportation modes and proposed a common faceted taxonomy based on facets of human-automation interface, environmental complexity, system complexity and societal acceptance. [Mostafa 2019] points out that the level of autonomy may be dynamically adjustable, given some situational awareness. In their literature review of levels of automation, [Vagia 2016] noted that some authors refered to “autonomy” and “automation” interchangeably, and proposed an 8-level taxonomy for levels of automation:
- Level 1 – Manual control
- Level 2 – decision Proposal
- Level 3 – human Selection of decision
- Level 4 – computer selects decision with human approval
- Level 5 – computer executes selected decision and informs the human
- Level 6 – computer executes selected decision and informs the human only if asked
- Level 7 – computer executes selected decision and informs the human only if it decides to
- Level 8 – autonomous control informs operators only if out of specification error conditions occur.
This consolidated view of an autonomous mobility taxonomy is defined and structured as in terms of the decision role taken by the computer system.
Taxonomies provide a means to classify instances, but the intended uses of the categorization limit the value it provides. For example, the [SAE 2018] classification may be useful in setting consumer expectations, and the developers of the other taxonomies proposed no doubt had other purposes in mind during their creation. One consideration is whether the proposed categorizations are useful in terms of the intended benefits from implementing some form of autonomy. [Araguz 2019] identified the need for (or benefits from) autonomy from the literature as improving performance in systems with high uncertainties; ameliorating responsiveness, flexibility and adaptability in dynamic contexts; and ultimately handling complexity (especially in large-scale systems), and in the aerospace field as a means to improve reliability and tolerance to failures. Defining a level of autonomy taxonomy based on the amount of operator control required, or the complexity of the scenario/ computational tasks arguably speaks toward those dimensions of intended benefits.
Presenting levels of autonomy as a numbered sequence of levels implies not just a classification taxonomy, but some quantification of an autonomy continuum, rather than a set of discrete and non-contiguous classification buckets. Most of these taxonomy proposals also leave full autonomy as a speculative category awaiting future technology advancements (e.g., in Artificial Intelligence) and do not provide existence proofs that meet the category definition. While to some autonomy may imply some degree of implementation complexity, the converse is not always true; increasing levels of complexity do not necessarily lead to autonomy. Constructing an autonomy taxonomy based on the decision roles taken by the computing systems is more interesting because it focusses on the actions of the system itself rather than external factors (e.g., scenario complexity, other actors). The decision roles themselves, however, are discrete categories, rather than a linear scale; and are independent of the importance of the decisions being made.
[Myhre 2019] reviewed autonomy classifications for land and maritime environments and proposed that a system is considered autonomous if it can legally accept accountability for an operation thereby assuming the accountability that was previously held by either a human operator or another autonomous system; thus classifying systems as autonomous or not. With non-autonomous systems, liability generally lies with the operator; with autonomous systems, liability generally shifts back to the designer. The scope of accountability or liability in the event of some loss is often described by a monetary value. Insurance contracts are often used to protect against potential liabilities up to some specified monetary level. Monetary values have the advantage of providing a simple linear scale. A system that can accept a $1M liability is thus arguably more autonomous than one that could only accept a $1,000 liability.
References
[Araguz 2019] C. Araguz, et al. “A Design-Oriented Characterization Framework for Decentralized, Distributed, Autonomous Systems: The Nano-Satellite Swarm Case.” International Symposium on Circuits and Systems (ISCAS). IEEE, 2019.
[Clough 2002] B. Clough, “Metrics, schmetrics! How the heck do you determine a UAV’s autonomy anyway.” AIR FORCE RESEARCH LAB WRIGHT-PATTERSON AFB OH, 2002.
[Elhannouny 2019] E. Elhannouny, & D. Longman. Off-Road Vehicles Research Workshop: Summary Report. No. ANL-18/45. Argonne National Lab.(ANL), Argonne, IL (United States), 2019.
[FAA 2016] FAA “Operation and Certification of Small Unmanned Aircraft Systems”, 81 Fed. Reg. 42064, 42065-42066 (rule in effect 28 June 2016); 14 C.F.R. Part 107.
[Ferreira 2018] Ferreira, Fausto, et al. “Liability issues of unmanned surface vehicles.” OCEANS 2018 MTS/IEEE Charleston. IEEE, 2018.
[Gasser 2013] T.Gasser, et al. “Legal consequences of an increase in vehicle automation.” Bundesanstalt für Straßenwesen (2013).
[Huang 2015] H. Huang, et al. “A framework for autonomy levels for unmanned systems (ALFUS).” Proceedings of the AUVSI’s Unmanned Systems North America (2005): 849-863.
[IMO 2019] IMO Legal Committee, 106thSession,27-29 March 2019.
[Lantz 2016] J.Lantz, Letter to IMO in response to circular 3574, USCG, 16707/IMO/C 116, Jan 2016
[Mostafa 2019] S. Mostafa, et. al., “Adjustable autonomy: a systematic literature review.” Artificial Intelligence Review 51.2 (2019): 149-186.
[Myhre 2019] B. Myhre, et.al., “A responsibility-centered approach to defining levels of automation.” Journal of Physics: Conference Series. Vol. 1357. No. 1. IOP Publishing, 2019.
[NACDL 2019] National Association of Criminal Defense Lawyers, “Domestic Drones Information center” retrieved 12/31/2019.
[NFAS 2017] Norwegian Forum for Autonomous Ships “Definitions for Autonomous Merchant Ships” 2017
[SAE 2018] SAE, “Surface Vehicle Recommended Practice: Taxonomy and Definitions for Terms related to riving Automation Systems for On-Road Motor Vehicles” (Revised ) June 2018
[Taeihagh 2019] A. Taeihagh, & H. Lim. “Governing autonomous vehicles: emerging responses for safety, liability, privacy, cybersecurity, and industry risks.” Transport reviews 39.1 (2019): 103-128.
[Vagia 2016] M. Vagia, et.al., “A literature review on the levels of automation during the years. What are the different taxonomies that have been proposed?.” Applied ergonomics 53 (2016): 190-202.
[Vagia 2019] M. Vagia, & O. Rødseth. “A taxonomy for autonomous vehicles for different transportation modes.” Journal of Physics: Conference Series. Vol. 1357. No. 1. IOP Publishing, 2019.